Hyperspectral Imaging: What is it?
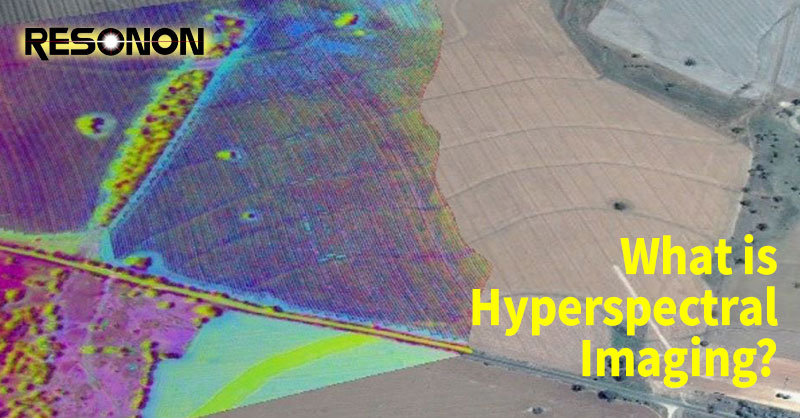
When compared to conventional imaging — such as a color camera — hyperspectral imaging yields more accurate color and material identification. It does this by providing far more detailed information for each pixel.
A color camera offers only three channels. In contrast, a hyperspectral camera divides the light signal into many tens to hundreds of bands or channels. This additional resolution improves machine vision accuracy, often dramatically.
Technology extended
Hyperspectral imaging is really a logical extension of conventional spectroscopy.
A spectrometer spreads a light beam into a continuous band of “colors.” For example, think of a prism. Taken together, the bands of colors are a spectrum of the light beam. Spectroscopy is the study or use of light spectra.
A hyperspectral imager acts like hundreds of spectrometers in parallel. This provides a spectral curve for each pixel in a scene (indicated schematically below).
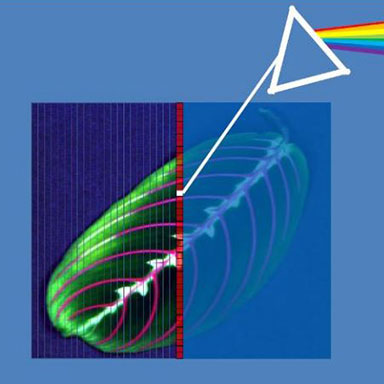
Why is it useful?
In contrast to the human eye, which captures only three primary colors, computer vision systems utilize many more color channels.
As an example, consider the color image of two types of candy shown below.
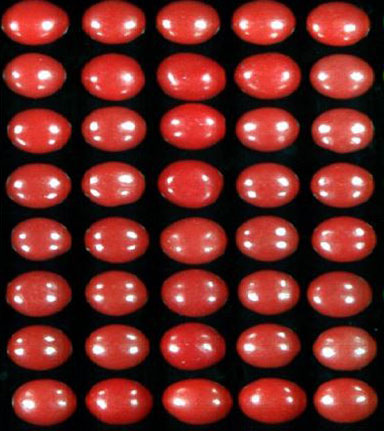
The position of one type of candy is in the shape of an “I.” A conventional color imaging system would have great difficulty discriminating between the two similarly colored candy types (as do many humans).
Show below are spectral curves for the two types of candy.
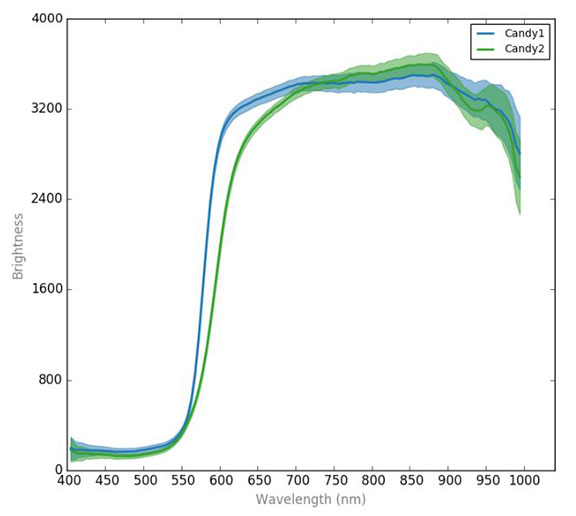
As one would expect with similarly colored objects, there is considerable overlap in the curves. However, there is also a region where there is a clear difference.
Machine learning algorithms readily exploit the difference in the spectral curves. The difference helps accurately classify the candy types — shown in a false color classification map. The false colors match the color of the curves.
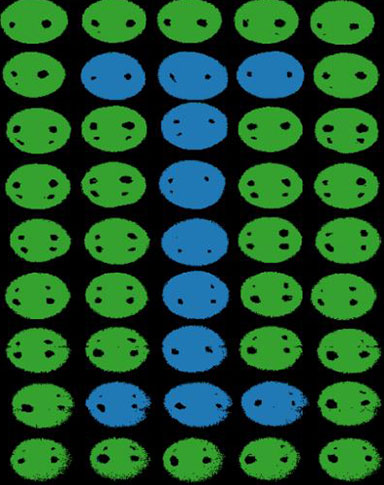
Hyperspectral data accurately differentiates the candy types. The classification is performed pixel-by-pixel. Note, almost every non-glare pixel is correctly classified.
Hyperspectral difference
Hyperspectral imaging provided more information per pixel. This information helps distinguishing between similarly colored objects or materials.
Users can interface outputs to robots, air-jets, labeling devices, etc.
Much like the human eye, hyperspectral imaging can apply to a wide range of applications. Such applications include: quality control (lumber, textiles, paper, building materials, drugs), process control (thin films, moisture content, color), sorting (food, recyclable materials, minerals), remote sensing (ocean color, environmental monitoring, agriculture), and more.
Today, hyperspectral technology is on platforms ranging from microscopes to airplanes — including UAVs.
Resonon offers a line of compact, low-cost, rugged hyperspectral imaging systems. The Pika-L, for example, integrates on to many UAV platforms.
Article provided by Resonon.